
The basics of Google Search and ranking
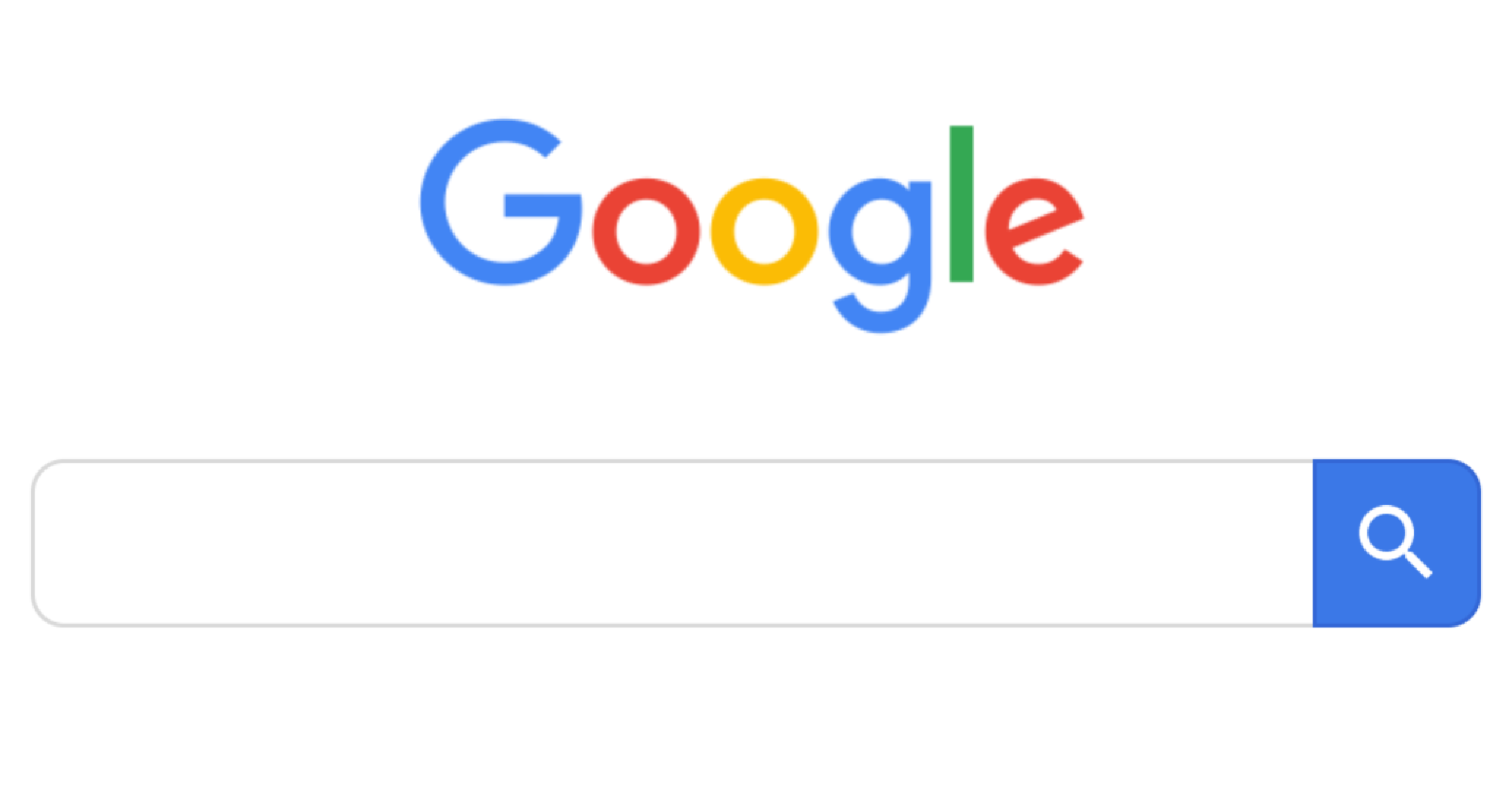
Google indexing
Google scans the internet and generates a duplicate known as an index. To illustrate, envision an index found at the conclusion of a book. Traditional search engines function in a comparable manner, searching for web documents.
However, the online landscape is dynamic. Nayak emphasized that sheer volume is not the sole factor, pointing out the considerable redundancy on the web. Google aims to establish an all-encompassing index.
As of 2020, Nayak estimated the index to be around 400 billion documents, though there was a period during which this figure declined, with the exact timeframe remaining unclear.
Google ranking
Google utilizes the index to fetch pages that align with a given query. The challenge arises from the fact that numerous documents could potentially "match" various queries.
Addressing this issue, a 2021 blog post by Nayak highlighted that Google employs "hundreds of algorithms and machine learning models," none of which depend entirely on a single, extensive model. Nayak clarified that these algorithms and models essentially sift through the index to identify the most pertinent documents.
Ranking signals
Several years ago, Google used to assert that it relied on over 200 signals for page ranking. In 2010, this number briefly surged to 10,000 ranking factors, with Google's Matt Cutts explaining that many of the initial 200+ signals had over 50 variations within a single factor--a detail often overlooked.
Now, as per Nayak's testimony, the count of Google signals has reduced to "maybe over a hundred." Notably, Nayak emphasized that, perhaps, the most crucial signal for retrieving documents aligns with what Google's Gary Illyes mentioned at Pubcon this year--the document itself.
According to Nayak, these key signals are:
- The document
- Topicality.
- Page quality.
- Reliability.
- Localization.
- Navboost.
Google core algorithms
Google employs core algorithms to narrow down the matches for a query to "several hundred" documents, assigning initial rankings or scores to these documents.
Every page that corresponds to a query receives a score. Subsequently, Google organizes these scores, utilizing them in part to present results to the user.
The scoring of web results involves an Information Retrieval (IR) score.
Navboost system
Navboost, as per Nayak, is deemed "one of the important signals" within Google. This "core system" specifically targets web results and isn't featured in Google's guide to ranking systems. It is also identified as a memorization system.
The Navboost system undergoes training using user data, memorizing all clicks on queries within the past 13 months (previously 18 months before 2017). According to Nayak, this system has been in existence since at least 2005, if not earlier. It has undergone updates over the years, evolving from its initial introduction.
Deep learning
Nayak stated that Google began incorporating deep learning in 2015, coinciding with the launch of RankBrain.
After obtaining a reduced set of documents, deep learning comes into play to fine-tune document scores.
Certain deep learning systems, like RankEmbed, play a role in the retrieval process, although the majority of this process occurs within the core system. Google uses three main deep learning models: RankBrain, DeepRank, and RankEmbed BERT.
